
According to the US EPA, municipal solid waste (MSW) landfills emit an estimated 4.5 million metric tons of methane (CH4) annually, making the industry the third largest methane emissions source in the United States. These methane emissions are approximately equivalent to the greenhouse gas (GHG) emissions from 30 million gasoline-powered passenger vehicles driven annually. Methane emissions from this industry are harmful to our environment and represent lost opportunity for the industry since they could otherwise be captured and converted to energy for revenue.
The industry and regulators are evaluating and adopting a wide variety of new methods and technologies to better detect, and ultimately mitigate, methane emissions. While rovers, quadruped robots and other technologies are being evaluated for efficient methane emissions detection, the most common automation systems in use today are sensors mounted on unmanned aerial vehicles (UAVs), commonly referred to as aerial drones. Three primary sensor technologies and methods are being used with varying degrees of success, each presenting different benefits and challenges for the application. The information as presented here provides details of three drone methods used for landfill emissions monitoring.
But, before reviewing the drone systems and their tradeoffs, let's start with a brief review of the currently accepted method for monitoring landfill emissions.
Surface Emissions Monitoring (SEM) for Landfill Methane Emissions
Surface Emissions Monitoring (SEM) was derived from a method commonly used in the oil and gas industry for leak detection. Applying this method of leak detection to the solid waste industry defines a leak source based on concentration where the landfill surface is assumed equivalent to the “interface periphery”.
The US EPA requires regular monitoring of landfill methane emissions according to US EPA Method 21, New Source Performance Standards (NSPS) Subparts AAAA and OOO, or other legacy regulations. It is important to note that while US EPA regulations have been adopted by most states, some states have instituted additional and more stringent monitoring rules for this purpose.
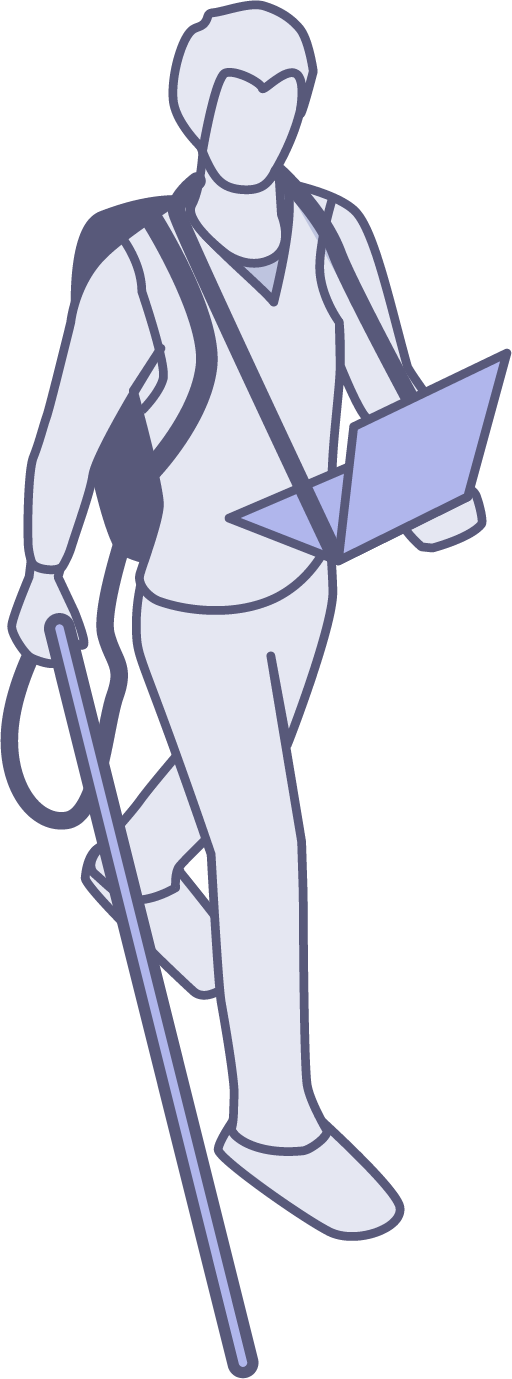
Generally speaking, the regulations stipulate that a technician walk the landfill surface within the waste boundary with a handheld point source leak detector along a sampling route – a serpentine path that traverses the landfill at no more than 30-meter intervals. The technician is required to take methane measurements along the sampling route, with the sensor probe located 5-10 centimeters (2-4 inches) from the landfill surface, to identify and isolate leak sources (Figure 1). Additionally, the inspection of the site must be performed at each cover penetration (gas wells, clean-outs, etc.) within the waste boundary, around the perimeter of the collection area, where visual observations indicate elevated concentrations of landfill gas such as distressed vegetation, at cracks or seeps in the landfill cover system, and at all cover penetrations. Leak locations where measured methane concentrations meet or exceed 500 ppm (parts-per-million) above background are required to be reported and remediated.
Method 21, which requires methane measurement within inches of the surface, turns out to be a good fit for landfill emission monitoring since landfill gas is heavier than air as a solution composed of CO2, CH4, mercaptans, and other volatile organic compounds (VOCs). Thus, the high-density of landfill gas compared to ambient air causes it to persist in landfill vegetation, especially when vegetation remains unmanicured.
For a 100-acre landfill, a technician will walk more than 20 miles over several days to perform a 30-meter serpentine inspection, including penetration and cover inspection.
Practically-speaking, however, Method 21 SEM for landfill emissions monitoring is onerous to implement and can be characterized as hazardous and labor-intensive. For instance, a 100 acre landfill surveyed over several days requires that a technician walk more than 20 miles to perform a 30-meter serpentine inspection including penetration and cover inspection. The landfill surface coverage is dependent on the chosen survey path, which can vary significantly between surveys, and also produces inconsistent results.
The ultimate objective of landfill SEM is to identify specific methane leak sources for mitigation by landfill technicians. Due to the nature of landfill operations and construction, the most common leak sources are fissures in the soil or geomembrane cover tears designed to minimize landfill gas from escaping. Common remedies including additional soil cover, regrading, geomembrane repair, gas system "tuning" (i.e. pulling greater vacuum), and others. In all cases, and as required by regulations, the leak source location must be marked and the location recorded. Generally, a flag is placed at the location as the marker.
For the reasons noted, much effort is being made by industry and regulators to develop alternatives to automate the SEM process to identify landfill methane leak sources more efficiently. As we review drone solutions for this purpose, consider the ultimate objective – find leak sources quickly and efficiently to aid in their repair.
Drone Methane Emissions Landfill Survey Techniques
Drones are an effective industrial tool to survey large areas, including landfills, and offer safety and efficiency benefits for a variety of use cases. This is particularly true for landfill methane emissions monitoring, and several techniques have been advocated for leak detection, source localization, and emissions rate determination (e.g., Fosco et al., 2024). Three of the most common drone systems used for landfill emissions monitoring are represented graphically (Figure 2).

All three drone solutions measure the concentration of methane, either at the landfill surface (Drone SEM) or within the plume resulting from landfill gas leakage (Ambient Air or Path Integrated measurement methods). Each method has benefits for methane emissions detection and quantification as summarized (Table 1) and described in further detail.
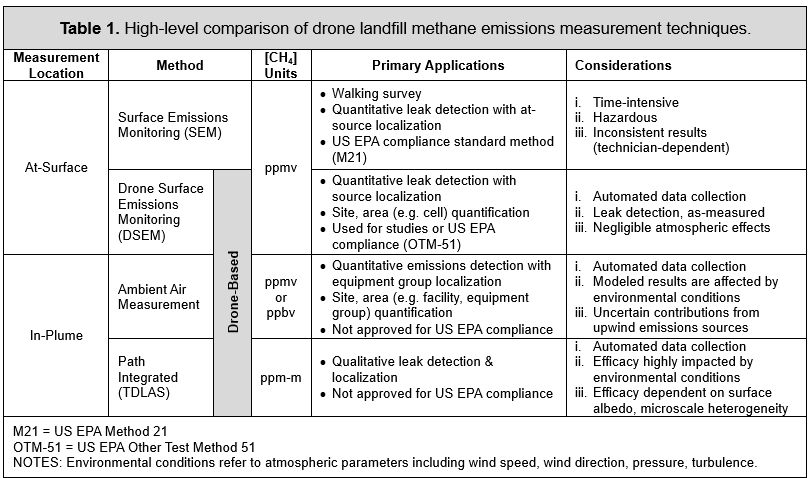
Drone Surface Emissions Monitoring (DSEM) System

The DSEM system was designed to automate the Method 21 SEM method to provide a safer, more effective, and efficient method for landfill surface emissions monitoring.
The DSEM approach utilizes a multirotor unmanned aerial system (UAS), flown at a constant height above ground level (AGL), while an umbilical allows for methane concentration measurements during flight from air samples taken directly from the landfill surface (Figure 3). A weighted air nozzle attached to the end of the umbilical captures gas that has pooled in the vegetation and surface irregularities of the landfill, providing a direct measurement of methane concentrations at the point of emission.
Both the SEM and DSEM methods measure the methane concentration in air samples in parts-per-million by volume (ppmv) measured within inches of the landfill surface and are effective at pinpointing leak locations without employing atmospheric models for interpretation. This direct measurement of landfill gas at the landfill surface directly identifies specific locations of leakage. The system also minimizes the impact of stochastic parameters by maintaining sampling directly at the landfill surface, where the gas concentration is least affected by environmental factors, leading to more accurate and reliable data.
Sniffer Robotics developed the DSEM method when it brought the SnifferDRONE™ CH4 UAS to market in 2020. In December 2022, the US EPA approved the use of the DSEM method for landfill methane emissions compliance (using the SnifferDRONE CH4) as an alternative to Method 21 as described in this announcement. The SnifferDRONE DSEM method and algorithm for quantifying emissions from DSEM data are detailed in the published EPA alternative method (OTM-51) and a contemporary peer reviewed publication (Abichou et al. 2023), respectively.
Ambient Air Measurements System
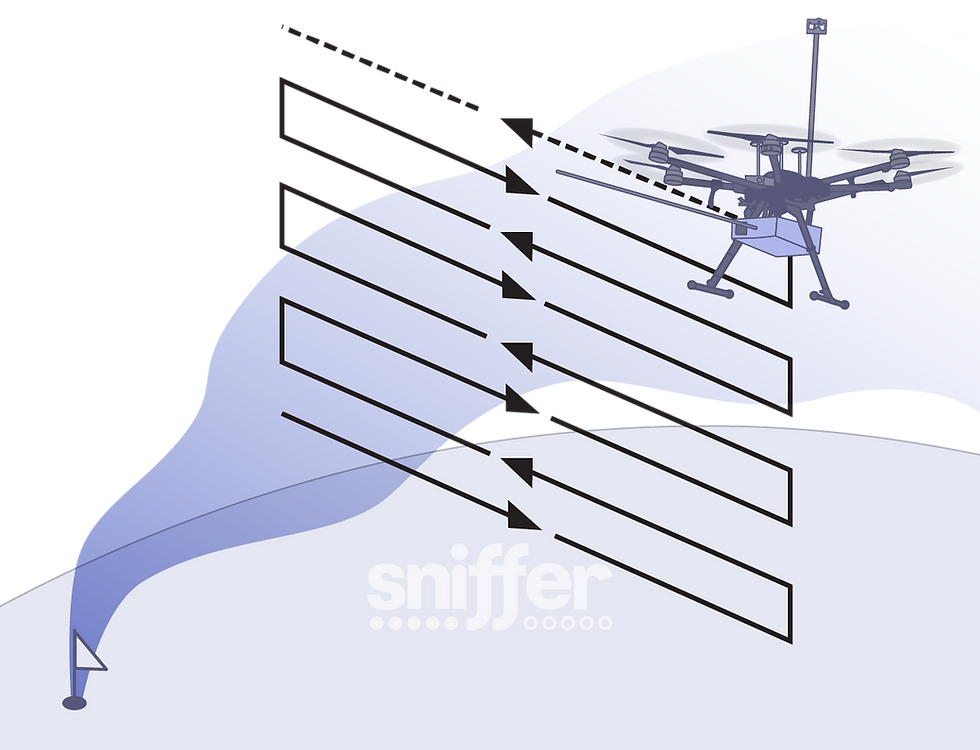
This sensor method can be effective for measuring total methane concentration enhancements within a plume that are sourced from an area in units of parts-per-billion (or million) by volume (ppbv or ppmv). The sensor configuration involves a methane gas sensor mounted on a drone in any of a variety of configurations to directly sample the gas at specific locations in 3D space, i.e. measurement from exactly where the drone is located (Figure 4).
Typical Ambient Air Measurements systems measure methane concentration by flying downwind of a specific source, area, or site to quantify the methane concentration distribution in a vertical flux plane (VFP) downwind of this area of interest (AOI). A VFP is also typically flown upwind of the AOI to understand potential contamination that might be captured in the downwind methane concentration data. The difference between the upwind and downwind flights should provide an accurate estimate of the total methane concentration enhancements sourced from the AOI.
This sensor approach is highly susceptible to environmental conditions. For source localization, the approach is less effective as it samples in-plume methane concentrations that are some distance away from the source. Therefore, identification of accurate source locations is highly dependent on atmospheric inverse plume modeling which is impacted significantly by wind, pressure, and turbulence. These environmental factors can introduce a significant amount of localization uncertainty using such in-plume Ambient Air Measurement data, uncertainties which increase with variable wind speed or direction. This sensing approach can be effective for concentration measurement of emissions from a known emitter, which can be a specific site or facility, because the VFP approach interrogates vertical methane concentrations downwind of the entire infrastructure.
Path Integrated (TDLAS) Sensor System
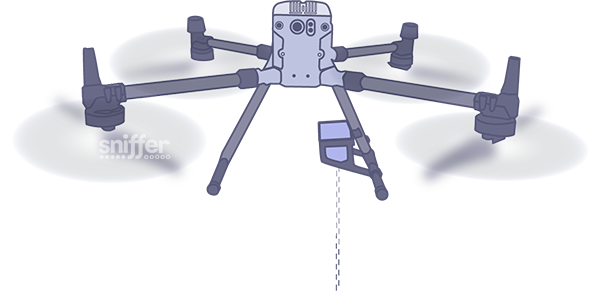
The laser sensor drone approach, also known as open-path Tunable Diode Laser Absorption Spectroscopy (TDLAS), utilizes a nadir-mounted laser source tuned to a specific methane absorption wavelength and a detector. Methane open-path TDLAS typically operate in the non-visible shortwave infrared region around 1.6µm where methane absorption features are moderate and water-vapor interference is minimized. A collimated laser is emitted from the drone downward towards ground and a fraction of the energy reflects off the surface back to the drone, where the depth of the methane absorption feature can provide an estimate of the methane concentration enhancement integrated across the entire laser pathlength. This measurement, known as an integrated concentration pathlength (CPL) measurement, is reported in units of parts per million-meter methane, commonly referred to as “ppm-m” of methane (Figure 5).
There can be some ambiguity in the deterministic nature of open-path TDLAS results. As an example, if a TDLAS laser sensor measured 100 ppm-m methane collected at 20-meters height above ground level, this could be caused by either of the following: (a) a dispersed plume away from source with a plume height of 10-meters and a methane concentration of 10 ppmv, or (b) a plume close to source 1-meter in height with a concentration of 100 ppmv. Thus it is helpful to have more information on the plume characteristics to effectively interpret ppm-m measurements with respect to identification of source locations, especially when TDLAS sampling is too sparse.
Analysis of the open-path TDLAS method reveals several outstanding technical challenges for this measurement approach:
Environmental Sensitivity: Similar to Ambient Air Measurement systems discussed above, variable atmospheric conditions, like wind speed and direction, can make atmospheric inverse plume modeling challenging. This can lead to significant uncertainty in the estimation of source locations and non-reproducible data.
Altitude Dependency: The path-integrated concentration measurements (ppm-m) are sensitive to altitude, making it essential to have the exact height above ground level (AGL) for every measurement point. On landfills, the terrain and ground cover varieties (e.g. tall grass) can make precision altitude measurement challenging and negatively affect data quality.
Source Location Interpretation Challenges: As described in the example above, ppm-m concentration measurements do not directly correlate with methane concentration by volume (e.g. ppmv) close to or away from the source. While it is reasonable to predict that the source location of a ppm-m plume map is close to the area where the highest value is determined, this is not always true. Localized areas of high ppm-m concentrations could result from episodic releases from infrastructure, such as valve actuators, or from dynamic atmospheric conditions (e.g., swirling winds). Thus, a specific ppm-m measurement is non-deterministic as a proxy for distance from source.
Sensor Performance due to Surface Albedo Variability: TDLAS systems inherently rely on the reflectivity of the surface, and different surface materials exhibit intrinsically different absorption characteristics. Due to the low altitude of the drone flights and high-spatial sampling, microscale surface heterogeneities can negatively impact TDLAS measurement. Absorption and scattering of laser photons by the surface can lead to weak signal-to-noise ratio (SNR) at the detector. Furthermore, any moisture such as standing water, snow, or even dew drops can negate any return of the reflected TDLAS signal. Because the surface of landfills varies widely between dirt, grass, and geomembrane, these albedo impacts on the TDLAS measurement must be well characterized before TDLAS methods are recognized for anything more than a qualitative method. Even by employing wavelength modulation approaches, uncertainties about TDLAS performance over different surface types and compositions remain.
Data Quality and Filtering / Rejection: Data quality flags are included in the TDLAS Laser sensor metadata to identify poor-quality data versus robust data. The derivation of these performance flags is inconsistent amongst TDLAS manufacturers. Without applying such filtering, which is typically a customized method in the data processing chain, TDLAS systems will suffer from an abundance of false negatives. While TDLAS detectors attempt to correct for surface absorption variability, the efficacy of these corrections is poorly characterized.
The issues highlighted above demonstrate inherent challenges and limitations of using TDLAS systems for methane emission monitoring at landfills. Given these uncertainties, open-path TDLAS can provide qualitative data at best, but concerns remain on the prevalence of false negatives. Actual data from field and controlled release studies provide further insight into the actual performance of these systems by characterizing TDLAS system performance (Table 2).
Table 2. Select contemporary studies on TDLAS laser sensor efficacy.
Year | Authors | Title | Publication |
2024 | Burgess, D. and Petsch, J. | Proceedings of the 2024 Canada SWANA Conference | |
2024 | Hossain, R., Dudak, Y., Buntov, P., et al. | CanCH4 symposium presentation, Ottawa, Canada, May 2024. | |
2022 | Sniffer Robotics & Major Waste Company | Methane Emissions Methods Comparison: SnifferDRONE | An intercomparison between three independent measurement techniques: DSEM, SEM, and TDLAS data |
Key takeaways from these studies include:
A Comcor-funded study, conducted by Burgess & Petsch (2024) at three landfills in Canada, observed no correlation between the locations of high methane concentration identified by traditional Method 21 SEM (the status quo) and those identified by drone-based TDLAS measurement.
Preliminary results from the St. Francis Xavier University’s Flux Lab 2023 Petrolia Controlled Release Experiment, presented by Hossain et al. (2024), demonstrated poor performance for leak detection efficacy from two different TDLAS drone systems.

Work with a major waste company, conducted by Sniffer Robotics (2022) and partners, evaluated the relative performance between DSEM, open-path TDLAS, and Method 21 SEM. The data showed strong correlation between DSEM and SEM results, but the TDLAS sensor data did not correlate with either (Figure 6).
Before the technical questions regarding TDLAS laser sensor performance can be systematically evaluated and understood, there is no justification that these sensors should be viewed as reliable for routine compliance monitoring. Similarly, the data analysis procedures must be normalized so that the data quality is consistent across the data produced by TDLAS systems in the field by any service provider.
DSEM Versus Other Drone Approaches

The Ambient Air Measurement and TDLAS laser sensor methods interrogate in-plume methane concentrations from landfill gas dispersion from a single or group of sources. Both of these in-plume measurement approaches are subject to influence from stochastic environmental parameters because they are taken above the landfill surface versus at-ground. The efficacy of either of these methods to estimate an emission source location is compromised by wind speed and direction. The dispersion of landfill gas is extremely difficult to model, difficulty that is compounded by unpredictable wind flow fields characteristic of landfills. Another difficulty that these complex wind conditions create are the lack of ability to execute flight paths in directions perpendicular to the wind to minimize the effects of double sampling. However, an evaluation of wind vectors across a typical landfill reveals the stochastic nature of winds across a landfill surface (Figure 7), which make atmospheric plume inverse modeling and perpendicular flight path execution nearly impossible.
A common issue with the Ambient Air Measurement and open-path TDLAS methods are that both rely on the data analysis method(s) as much as the measurement technology. The analytical methods applied downstream of data collection with either sensor can demonstrate markedly different results even with identical datasets. Without a higher fidelity effort to normalize the measurement performance and analytical methods for area source localization, neither Ambient Air Measurement nor open-path TDLAS shows potential to be approved for compliance.
Accuracy Matters! As the industry adopts the use of new technologies for landfill emissions monitoring, a focus should be placed on using technologies, systems, and methods that are not prone to false positives and false negatives - false negatives being the most problematic since they are informing there are no emissions, when in fact there are.
Conclusion
Sniffer Robotics is focused on providing the industry's most effective and efficient technology for the benefit of precisely and accurately detecting and accounting for landfill methane emissions. We are sharing this brief to educate customers as to different systems that are in use for drone-based landfill methane emissions measurements, including several fundamental differences in the measurement and detection methods.
While Sniffer Robotics commercial solution for drone-based emissions monitoring is the SnifferDRONE, the company continues to invest in research and development while evaluating alternative technologies and methods. For example, Sniffer Robotics owns multiple TDLAS sensors and continues to evaluate their attributes and performance characteristics. However, to date, we have not yet found any other drone-based sensor technology or method capable of producing reliable or repeatable results comparable to the DSEM method.
Please contact us if you have any questions regarding this technical brief and/or for further information about our solutions that help improve operations and our environment.
References
Abichou, T., Bel Hadj Ali, N., Amankwah, S., Green, R., and Howarth, E.S. (2023) Using Ground- and Drone-Based Surface Emission Monitoring Data to Locate and Infer Landfill Methane Emissions. Methane 2(4), 440-451.
Burgess, D. and Petsch, J. (2024) Identifying Methane Surface Emissions – A Comparison of Field Method Approaches. Proceedings of the 2024 Canada SWANA Conference, Niagara Falls, Canada, April 2024.
Fosco, D., De Molfetta, M., Renzulli, P., and Notarnicola, B. (2024) Progress in monitoring methane emissions from landfills using drones: an overview of the last ten years. Sci. Total Environ. 945, 173981.
Hossain, R., Dudak, Y., Buntov, P., Canning, E., Martino, R., Fougère, C., Naseridoust, S., Bourlon, E., Lavoie, M., Khaleghi, A., Farjami, F., Ells, L., Berthiaume, M., Hall, C., and Risk, D. (2024) A Controlled Release Experiment for Investigating Methane Measurement Performance at Landfills.
Commentaires